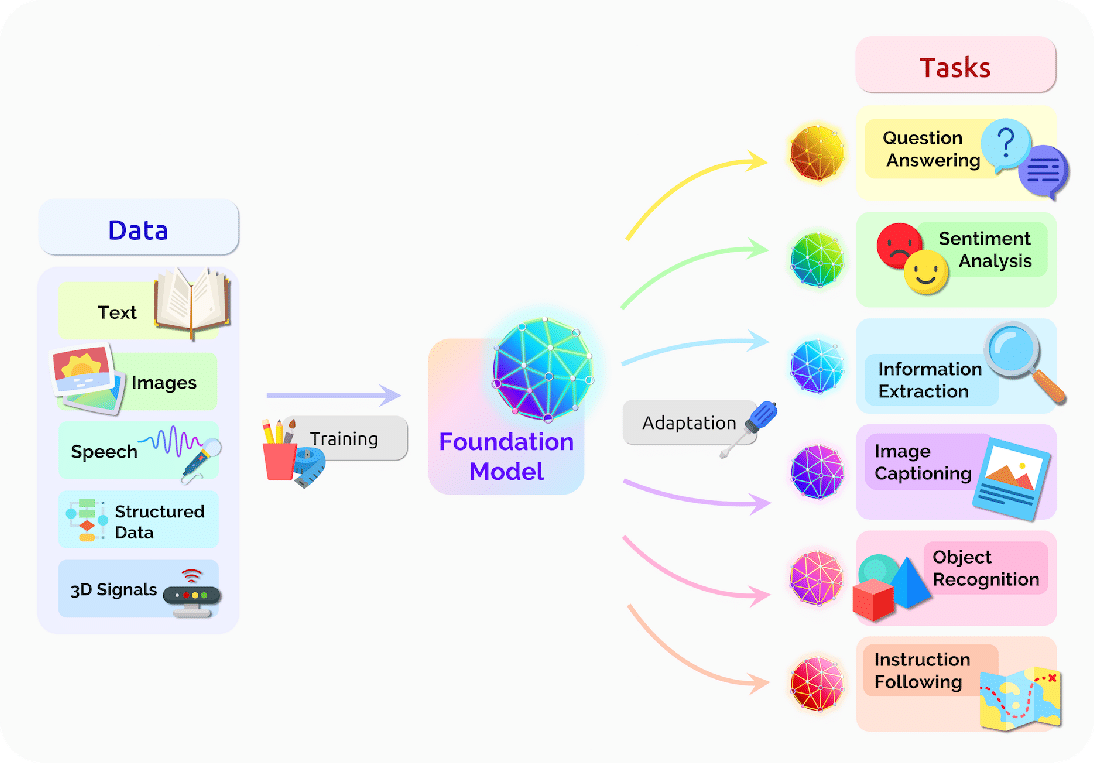
Just over one year ago, OpenAI introduced ChatGPT, a tool that harnesses artificial intelligence (AI) to engage in human-like conversations—answering questions, composing emails, and coding. Since its introduction, this tool has generated considerable attention for its impressive achievements, including passing law, business, and medical school exams, and tackling complex programming queries. Likewise, ChatGPT has also raised significant concerns, such as facilitating the creation and dissemination of misinformation, exhibiting biases, and making student cheating on exams easier.
But what should we consider as ChatGPT enters its second year? In this week’s post, we will explore this question and offer two things you should pay attention to over this year.
Increasing Focus on Foundation Models
Most discussions about ChatGPT focus on it as a standalone application or as something that integrates with other products, such as Khan Academy’s tutoring tool, Khanmigo. While these views of ChatGPT capture most of its uses, ChatGPT is also an example of a robust model that can serve as the core of an artificial intelligence infrastructure. This type of model, called a “foundation model,” trains on large amounts of content (text, images, etc.) and can adapt to perform a wide range of downstream tasks, such as object recognition or information extraction (Figure 1).
One key component of a foundation model is “transfer learning,” where the knowledge obtained from training transfers to downstream tasks or from one task (object recognition, for example) to another, such as information extraction. Improving this transfer learning requires robust computing power, such as promised by the partnership of Amazon and Anthropic, and increased training data (extending the knowledge cutoff date in OpenAI from 2021 to 2024).
While foundation models like ChatGPT present risks, we expect them to become more prevalent in the coming year. As a result, we see ChatGPT as becoming more than an application that we use on its own or as part of another piece of technology, but instead, as an engine that powers an entire artificial system that looks to address real-world tasks.
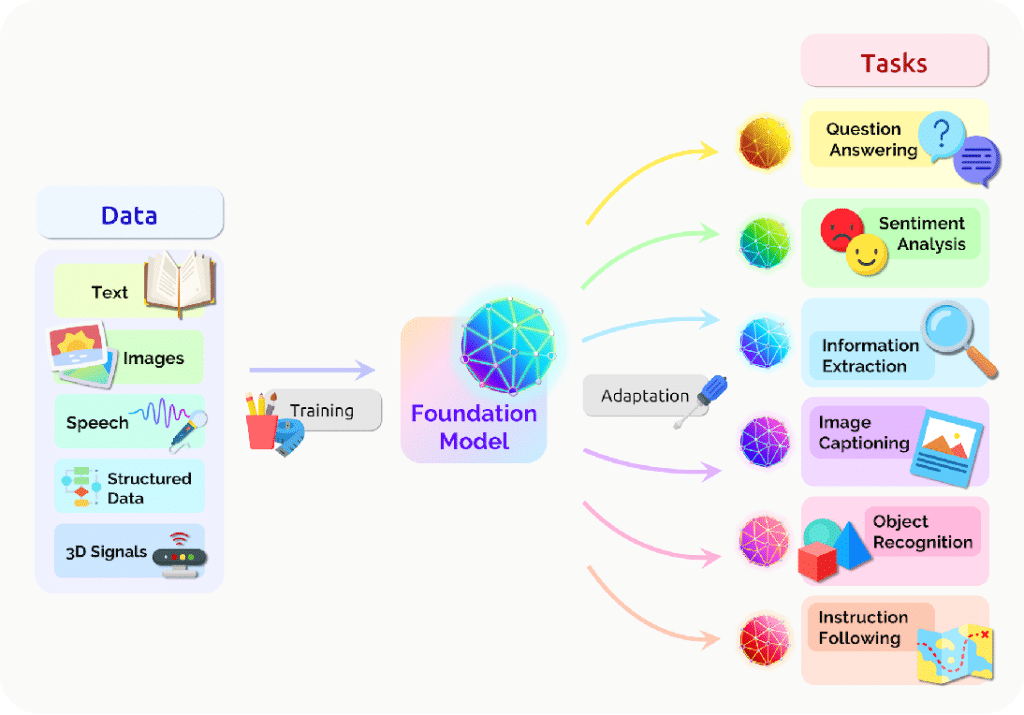
Continued Questions about “Openness”
The founder of OpenAI initially described their company’s mission as “to advance digital intelligence in the way that is most likely to benefit humanity as a whole, unconstrained by a need to generate financial return.” This mission statement (and the company’s name) suggested that the technology would be transparent, reusable, and extensible enough to allow developers to build ChatGPT-powered applications.
However, since 2019, Open AI itself shifted from a non-profit to a for-profit. Also, in 2020, the company restricted full access to the GPT-3 model, an earlier version of the current ChatGPT, to Microsoft. Finally, OpenAI restricted free use access to its AI-powered text-to-image tool, DALL.E, and replaced it with a freemium offering. As a result, the “openness” of OpenAI has been cast into doubt.
However, according to some recent research, the notion of “openness” in artificial intelligence is problematic. For example, any developer seeking to develop an artificial intelligence tool to compete with ChatGPT would need access to training data, software to build the tool, and computing power to train it. However, the authors argue that this is nearly impossible as access to training data is often kept secret, large corporations usually control the software required to build such models, and the computing power to train models is beyond the reach of any typical developer or company.
Summary
Of course, the coming year will involve discussions about how institutional leaders might consider deploying artificial intelligence in general and Chat GPT in particular at their institutions. For example, some organizations, such as College Complete America, have recently released playbooks to guide leaders through this process. Likewise, there will be a concentration on the impact of new Chat GPT functionality, such as the rumored “Q* Project,” which suggests Chat GPT may move into solving elementary math problems. Lastly, we will hear more about efforts to address the risks of artificial intelligence by encouraging explainability and fairness in developing AI models.
However, while these are important focus areas, we assert that foundation models and the debate around “openness” have the greatest chance of changing the education technology landscape. Foundation models, for example, may result in the convergence of technology in education, as many solutions resting on a single model may combine downstream tasks into one solution, perhaps shrinking the technology landscape. Likewise, if “openness” occurs in artificial intelligence, we may see a democratization of AI development, with smaller vendors creating competitors to Chat GPT and other AI tools, which may increase the availability of technology available to institutional leaders.
We will monitor these two areas throughout the year and report our findings. Please reach out with feedback or questions.